The Intersection of Artificial Intelligence and Ophthalmology
XXX Congreso Boliviano de Oftalmología
Click me for more details
Click me for more details
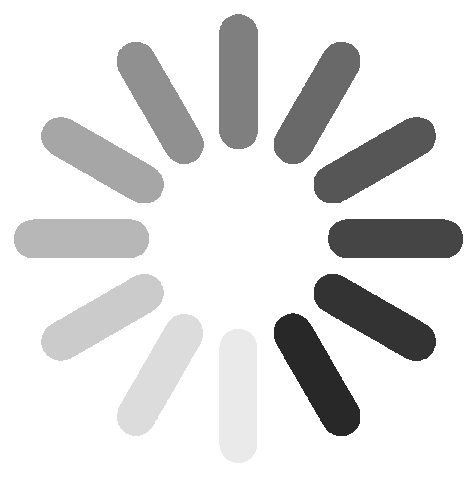
An alternative, and more general theme for the presentation is the rapid exploration of large research spaces. That is, how we can use artificial intelligence and data analysis techniques to take many thousands, if not hundreds of thousands of research papers, and rapidly understand the themes and topics they discuss.
Within this deeper context then, we can explore the research space where artificial intelligence meets ophthalmology. To understand the current state of research. And then to consider where research might head in the future.
How is artificial intelligence used in ophthalmology?
My aim is for us to embark together on a journey of discovery. All of us here belong to one of two groups:
- those with experience in artificial intelligence - like me. or
- those with experience in ophthalmology - like most of you in the audience.
Despite our different academic backgrounds, our goals are the same; at this moment we all want the following:
- to understand how artificial intelligence is used in the field of ophthalmology.
- to obtain an insight into the current state-of-the-art in scientific research regarding where and how artificial intelligence is used in ophthalmology.
- and to appreciate the history of this research and use it to predict where the state-of-the-art will likely develop in the future.

Maximum of 200 unique words allowed.
Includes both single words and collocations (bigrams) of two words.
Click me for more details
Data Acquisition
We begin by performing a comprehensive search of the PubMed medical literature database. This search was defined to be as broad in scope as possible by deliberately specifing only three keywords: 'ophthalmology', 'artificial' and 'intelligence'. No additional search filters were applied.
By keeping the search terms general, we allowed for maximum flexibility in the results. For instance:
- The search terms were not restricted by punctuation or specific phrasing.
- No date range was imposed, allowing us to capture the full history of relevant research.
- The language of the articles was not restricted in any way.
- The terms could appear anywhere in the text - whether in the title, abstract, or keywords.
- We did not limit the search to specific MeSH (Medical Subject Headings) terms, nor did we filter by author-supplied keywords.

Date of search: 2024/08/23. Total number of articles: 3,913
Click me for more details
This wide-net approach was intentional. By deliberately keeping our search net as wide as possible, we aimed to capture a high-level panoramic view of as many relevant research articles as possible, within the context of artificial intelligence and ophthalmology, and regardless of specific subfields or publication details.
This approach gave us a better understanding of the broader landscape of AI applications in ophthalmology such that we could observe the wide range of research that has already been conducted, through to the current state of investigation, before then focusing on specific areas in later analyses.
This initial search returned 3,934 articles. Once we eliminated edge cases such as letters to the editors, responses, and research proposals, we were left with 3,913 articles for analysis.
A preliminary review of these articles reveals several key observations:
- Several articles were returned with a direct mention of "artificial intelligence" in the title or abstract, highlighted in red, showing the immediate relevance of these papers to our investigation.
- Many papers discussed ophthalmic diseases, conditions, or techniques, highlighted in yellow, where AI plays a critical role, highlighting its direct application in clinical settings.
- However, some articles had less obvious connections to either ophthalmology or artificial intelligence. These are highlighted in blue. That their relationship to the use of AI in ophthalmology is not so clear suggests they may be tangential to our primary focus. Certainly a deeper investigation would be needed to determine their relevance, which is precisely what we go on to perform below!
The first five articles from this search are listed below, with relevant keywords highlighted in the colours described above:
➞ Comparison of 21 artificial intelligence algorithms in automated diabetic retinopathy screening using handheld fundus camera.
➞ Glucocorticoid therapy for sepsis in the AI era: a survey on current and future approaches.
➞ Text mining of hypertension researches in the west Asia region: a 12-year trend analysis.
➞ Design, synthesis, and evaluation of cyclic C7-bridged monocarbonyl curcumin analogs containing an o-methoxy phenyl group as potential agents against gastric cancer.
➞ Artificial Intelligence to Facilitate Clinical Trial Recruitment in Age-Related Macular Degeneration.
Publications by Country
Loading plot...
Use the mouse to access the tools towards the top right of the chart.
Let's begin our analysis with a basic bibliometric overview. The bar chart above illustrates the distribution of publications, returned by our PubMed search on artificial intelligence in ophthalmology, grouped by country.
Immediately, we see that the United States and the United Kingdom dominate the landscape, together accounting for nearly 70% of the publications. This substantial contribution is followed by several European nations, including Switzerland, the Netherlands, and Germany. Interestingly, we also see representation from China and India, two major Asian economies who are also contributors to the artificial intelligence and ophthalmology field.
While this initial analysis provides a clear picture of where the majority of research is being produced, it also raises important questions about the potential risks of bias in the global research landscape. For example, the relative absence of publications from regions such as Latin America, Africa, and other parts of the world suggests there may be gaps in our understanding of how AI is being applied in ophthalmology outside of traditionally research-dominant countries. This geographic disparity might hint at the well-known 'North-South divide' in scientific research, where wealthier nations in the Northern Hemisphere often have more resources to conduct and publish research than those in the Southern Hemisphere.
As we move forward with our analysis, it is important to keep this potential bias in mind. Could it be that key research from certain regions is underrepresented in global databases like PubMed? Or, more critically, are there AI-driven advancements in ophthalmology occurring in these underrepresented areas that are not being captured? Or perhaps these Southern hemisphere regions missing out on using state-of-the-art artificial intelligence in their clinical practice? This is a crucial consideration, as any bias in the data could affect our conclusions about the current state and future trajectory of AI in ophthalmology.
Publications by Language
Loading plot...
Use the mouse to access the tools towards the top right of the chart.
Turning now to the analysis of publications by language, we observe a striking imbalance: nearly 98% of the papers are written in English. This overwhelming dominance reflects the status of English as the lingua franca of scientific research, particularly in global databases such as PubMed.
However, it is worth noting the relatively small number of publications in other languages. For example, only 4 papers were published in Spanish, despite it being one of the most widely spoken languages in the world. Of course this disparity may partly be explained by the fact that PubMed is a U.S.-based database, and English has long been the preferred language of international academic journals. Yet, the scarcity of non-English publications raises important questions about accessibility and inclusion in the global research landscape.
When scientific publications are overwhelmingly in one language, it can create barriers for non-English-speaking researchers and clinicians. This, in turn, may limit their ability to access, contribute to, or apply new knowledge in their own regions. The underrepresentation of other languages in these databases might also mean that valuable research from non-English-speaking countries is underreported, or that these countries are less likely to contribute to global discourse on topics such as AI in ophthalmology.
This language imbalance is not just a matter of convenience, but may reflect broader issues of equity and access in health care, health care based technology, and scientific communication overall. As we move forward, we should keep in mind how language might act as a barrier - not only in terms of accessing research but also in terms of contributing to it. Are there important studies in languages like Spanish, Chinese, or others that are being overlooked simply because they are underrepresented in databases such as PubMed? Does this also mean the health care technology (like AI in ophthalmology) is not accessible or being applied in these regions? How might we address this issue to foster a more inclusive, global approach to the dissemination of scientific knowledge?
Publications by Year
Loading plot...
Use the mouse to access the tools towards the top right of the chart.
When we examine the distribution of publications by year, we notice a clear trend: from 2018 onward, the pace of research in AI applied to ophthalmology accelerates dramatically.
This rapid growth can be attributed to several key factors:
- Technological advances in AI capabilities: Breakthroughs in machine learning algorithms, especially in deep learning, significantly enhanced the ability to process complex medical data, such as retinal images.
- Greater data availability: The increased adoption of electronic health records (EHRs) and large-scale medical imaging datasets made it possible to train AI models with unprecedented accuracy and scale.
- More powerful computing resources: The availability of high-performance GPUs and cloud-based computing platforms democratized access to the computational power required for large-scale AI experiments.
- Increased investment and interest: Both the public and private sectors recognized AI's potential to transform medicine, leading to greater funding for research and development.
- Broader recognition of AI's transformative potential in medicine: As AI applications, particularly in image analysis, proved effective in other fields, ophthalmology researchers began exploring its potential to revolutionize diagnosis, treatment, and patient outcomes.
From the chart we see that one of the most pivotal periods was from 2015 to 2018. During this time, advancements in AI (and equally the computational power available) enabled the processing of complex image data with greater accuracy and efficiency. This was particularly relevant to ophthalmology, where the analysis of retinal images and other diagnostic imaging became a prime area for AI-driven innovation. The growing public awareness of AI's capabilities in everyday technologies, such as facial recognition - widely popularized by Apple's introduction of Face ID with the iPhone X in 2017 - likely contributed to an increasing interest in AI applications in healthcare.
From 2018 onward, the combination of these factors created a fertile ground for AI research in ophthalmology, resulting in a marked increase in the number of publications. The data illustrates this clearly: by 2023, over 800 papers on AI in ophthalmology were published, compared to just 25 papers in 2015. This swift growth underscores the rapid development of AI technologies and their increasingly vital role in advancing ophthalmic care.
But that was quite superficial
Could artificial intelligence help us learn more?
Yes! Of course!
We can use AI itself to learn more about how AI is used in ophthalmology!
Panorama of Investigation
Loading plot...
Hover the cursor over the points for additional details.
Click on the legend to toggle the visibility of the clusters.
A more advanced way to understand the scope of the research papers returned to us by our PubMed search is to perform a type of data analysis known as topic modelling. This technique, which leverages artificial intelligence, allows us to automatically (and therefore rapidly) uncover the underlying structure of our research corpus by grouping articles based on their thematic content.
In this case, we applied topic modelling to the titles of all 3,913 articles returned from our PubMed search. Topic modelling is the core concern of the BERTopic library (by Maarten Grootendorst), which we used for this purpose. The analysis begins by creating vector embeddings — essentially, mathematical representations of the titles — using the SentenceTransformer library and the 'all-mpnet-base-v2' model, which maps each title to a 768-dimensional dense vector space. To make the data more manageable and useful for visualization, we then reduced the dimensionality of these vectors to two dimensions using the UMAP technique, for general non-linear dimension reduction.
Next, we applied HDBSCAN, a clustering method, to group the articles based on their proximity to one another in this newly reduced two-dimensional space. This proximity is used as a proxy for the semantic similarity of the titles within a particular cluster, and therefore each cluster is assumed to correspond to a distinct research topic. Finally, we used the TF-IDF approach, implemented with the sklearn CountVectorizer, to extract the most representative keywords from each cluster. These keywords were then passed through the Llama-2-13b-chat-hf large language model, supplied also with an appropriate prompt, to generate concise and meaningful topic labels.
The result of this process is the two-dimensional cluster plot shown above. Each point on the plot represents one of the research papers, and the colour coding reflects the highest-level topics that emerged from the analysis. As you can see, three broad topics dominate the research landscape:
- Retinal disease detection using artificial intelligence (in pale green).
- Robotic surgery in ophthalmology (in dark green).
- Diagnosis of retinopathy of prematurity (in wine colour).
What makes this analysis particularly powerful is its ability to summarize and organize a vast amount of information — almost 4,000 papers — in a matter of minutes. By using AI to understand how AI is applied in ophthalmology, we can efficiently map the key areas of research. No human, whether an intern or a seasoned researcher, could manually process and categorize these articles with such speed and precision.
This cluster plot acts as a 'map' of the research 'landscape', providing us with a quick and intuitive way to understand the current state of AI in ophthalmology. It allows us to see the major research topics at a glance and provides a clear framework for deeper exploration of specific areas of interest.
Word Embeddings
Representing words as numbers
Let's take a brief detour into some of the underlying the mathematics that makes topic modelling possible. To perform the type of analysis shown above, we first need a way to translate language - words and sentences - into a numerical form. After all, fundamentally a computer can only understand and manipulate numbers, therefore some form of conversion from text to numbers was inevitable. This process is known as embedding, and it allows us to represent words as vectors in a high-dimensional space.
To illustrate this, consider a simple example in two dimensions. Let's encode a set of familiar words using two very straightforward characteristics to give us a visual from which we can understand what is happening. We use the two features 'Is Royalty' and 'Is Masculine' and represent each word in our corpus in terms of these two features. In this way our vocabulary can be represented as a vector where these characteristics are either present (1) or absent (0).

"King"
Is Royalty: 1
Is masculine: 1

"Queen"
Is Royalty: 1
Is masculine: 0

"Man"
Is Royalty: 0
Is masculine: 1

"Woman"
Is Royalty: 0
Is masculine: 0
For example:
- King: has both attributes, so it is represented as (1, 1).
- Queen: is royalty but not masculine, so it is represented as (1, 0).
- Man: is masculine but not royalty, yielding (0, 1).
- Woman: has neither attribute, resulting in (0, 0).
These vectors provide a simple mathematical representation of each word, allowing us to visualize and quantify their relationships. We can see that King and Queen are more closely related than King and Man, as their vector difference reflects a smaller angular separation. This reflects the semantic similarity between words: words with similar meanings will have vectors that are closer together in the vector space.

Moreover, word embeddings enable us to perform arithmetic operations that reveal meaningful linguistic relationships. For instance, subtracting Queen from King yields a vector (0, 1), as does subtracting Woman from Man. This equivalency allows us to observe that the relationship between 'King' and 'Queen' is analogous to the relationship between 'Man' and 'Woman.' In this way, word embeddings encode semantic relationships, enabling computers to understand and process language with a degree of nuance.
"King" - "Queen" = (1, 1) - (1, 0) = (0, 1)
"Man" - "Woman" = (0, 1) - (0, 0) = (0, 1)
"King is to Queen as Man is to Woman"
In general, embeddings map words to a continuous vector space, where their relative positions encode various syntactic and semantic properties. As such, words that share similar meanings tend to cluster closely together, making embeddings a powerful tool for understanding and modelling language. We have codified a linguistic meaning, which allows us to state "The king is to the queen as the man is to the woman." And this is how we can encode meaning into words and sentences. In our topic modelling, embeddings capture the semantic content of research paper titles, allowing us to group papers by themes based on their position in the vector space. This underlying mathematical representation is the foundation upon which more advanced models, such as BERTopic, are built.
Word Embeddings
Representing words as numbers
One of the remarkable capabilities of artificial intelligence, particularly in natural language processing, is the ability to analyze language with increasing levels of complexity by adding more dimensions, or features, to our representations.
Continuing with our previous example, let’s introduce an additional dimension: Is Synonymous with Power.

"King"
Is Royalty: 1
Is masculine: 1
Is synonymous
with power: 1

"Queen"
Is Royalty: 1
Is masculine: 0
Is synonymous
with power: 1

"Man"
Is Royalty: 0
Is masculine: 1
Is synonymous
with power: 0

"Woman"
Is Royalty: 0
Is masculine: 0
Is synonymous
with power: 0

"Police"
Is Royalty: 0
Is masculine: 0
Is synonymous
with power: 1

"Kingdom"
Is Royalty: 1
Is masculine: 0
Is synonymous
with power: 1
With this new feature, we can further distinguish the semantic nuances of our words. For instance:
- King: becomes (1, 1, 1), as he is both royalty and masculine, and also synonymous with power.
- Queen: would now be represented as (1, 0, 1): she is royalty (1), not masculine (0), but associated with power (1).
- Man: would be (0, 1, 0): masculine but neither royal nor commonly associated with power.
- Woman: remains (0, 0, 0), as she does not fulfill any of the three characteristics here.
Indeed let's add two more words to our vocabulary: Police and Kingdom, and we can see how they fit into our new feature space.
- Police: would be (0, 0, 1): not royal, not masculine, but associated with power.
- Kingdom: would be (1, 0, 1): royal, not masculine, and associated with power.
As we add more dimensions to our vectors, we can capture increasingly subtle aspects of meaning. This is essential for nuanced fields like AI in ophthalmology, where precise distinctions are necessary to differentiate between complex concepts. The research paper titles from PubMed that we’re analyzing, for example, are embedded in a 768-dimensional vector space. Each of these dimensions encodes a distinct linguistic or conceptual feature, which allows the model to represent the full scope of ideas and themes within these titles.

The resulting embeddings provide an incredibly rich and high-resolution representation of meaning, enabling BERTopic to abstract and analyze relationships between terms at a level of detail that would be impossible with only a handful of features. As we increase the dimensionality of these embeddings (recall we are using the 'all-mpnet-base-v2' model, which maps each title to a 768-dimensional dense vector space) we are essentially expanding the model's ability to recognize and process the vast array of subtle relationships that exist between terms in specialized subject areas, such as our original goal "artificial intelligence in ophthalmology".
In this way, we can see how BERTopic can use our embeddings to understand the relationships between words and concepts, and in turn use these relationships to analyze and interpret the research paper titles it is given.
Panorama of Investigation
Loading plot...
Hover the cursor over the points for additional details.
Click on the legend to toggle the visibility of the clusters.
Having introduced the fundamentals of word and sentence embeddings, let's return to the scatterplot visualization we showed earlier, where each point represented one of the nearly 4,000 research paper titles from our PubMed search. Previously, we examined this scatterplot with a high-level topic model that identified three broad themes: Retinal Disease Detection Using Artificial Intelligence, Robotic Surgery in Ophthalmology, and Diagnosis of Retinopathy of Prematurity. We noted how this initial overview was partiularly powerful in it's ability to summarize and organize a vast amount of information - the almost 4,000 papers - thereby providing a snapshot of the major areas of AI application within ophthalmology.
Now, with a deeper understanding of the semantic encoding of these titles, we can delve further into the data. And so, in the second version of the scatterplot (see below) I've increased the number of distinct topics for BERTopic to find to 20. In doing so, we reveal an even more detailed view of the research landscape. This level of granularity is made possible by leveraging the 768-dimensional embeddings generated by the 'all-mpnet-base-v2' model, which capture a rich array of linguistic and semantic features. These high-dimensional embeddings allowed BERTopic to identify subtle relationships and thematic clusters that reflect nuanced variations across different areas of AI research in ophthalmology.
As always, each point on the scatterplot is placed based on the semantic content of its title, with similar topics grouped closely together. These clusters, which we refer to as topics (hence the word topic in the name BERTopic), emerge from the linguistic and contextual similarities encoded in the embeddings. This refined clustering highlights the diversity of AI applications in ophthalmology, enabling us to see the intricate patterns and specialized topics that would not be immediately apparent from a high-level analysis.
This layered approach demonstrates how AI can extract a wealth of information from textual data, allowing us to move beyond general themes and uncover the finer distinctions within the field.
Loading plot...
Hover the cursor over the points for additional details.
Click on the legend to toggle the visibility of the clusters.
Building upon the detailed 2D scatterplot with 20 distinct topics, we now move to an interactive 3D visualization. This plot allows for dynamic exploration — you can pan, rotate, and zoom in or out, enabling a more immersive way to examine the thematic structure of our research corpus.
Think of this 3D space as a navigable landscape of semantic relationships. Each point represents a research paper title, positioned based on the nuanced semantic similarities and differences uncovered by the 768-dimensional embeddings from the 'all-mpnet-base-v2' model. The interactive aspect of this plot allows you to delve deeper into specific clusters, visually tracing how topics relate to one another in this semantic space.
By navigating this landscape, you can observe how certain clusters form peaks of concentrated research areas, while others span broader, less dense regions, reflecting more generalized AI applications within ophthalmology. This visual and interactive approach provides a tangible sense of the diversity and interconnectedness of topics across the field, offering both high-level insights and the ability to focus on specific areas of interest.
Loading plot...
Hover the cursor over the points for additional details.
Click on the legend to toggle the visibility of the clusters.
The interactive 3D visualization not only enhances our understanding of the thematic structure but also exemplifies how advanced AI tools can transform vast, complex datasets into intuitive and engaging formats. This 'map' of the research landscape serves as a guide, revealing how AI is being applied in ophthalmology and helping us to see both the connections and the distinctions between different areas of research.
Evolution of Keywords and Key Phrases in Research Paper Titles
Loading plot...
In order to understand how the focus of research in AI applied to ophthalmology has evolved over time, we can analyze the frequency of key phrases, or n-grams, within the titles and abstracts (see next section) of the research papers returned by our PubMed query.
An n-gram is a contiguous sequence of n words in a text. For example, a single word is a 1-gram, a two-word phrase is a 2-gram, and so on. By identifying and tracking the most frequently occurring n-grams, we can gain insight into the dominant themes, specific techniques, and trending applications over the years.
The stacked bar chart above presents the evolution of the top 15 n-grams found in the titles of the research papers from 2018 to 2024. Each bar represents a year, with the width of the segments indicating the percentage occurrence of each n-gram relative to the total n-grams for that year.
Several trends are immediately noticeable:
- Artificial Intelligence and Deep Learning: these terms consistently appear in a significant portion of the titles each year, underscoring their foundational role in this body of research. However, their relative prevalence has fluctuated, indicating shifts in focus within the broader category of AI applications in ophthalmology.
- Optical Coherence Tomography (OCT): terms related to OCT appear frequently, reflecting the importance of this imaging technology in ophthalmic research. As the technology has become more integrated with AI, we see recurring mentions in conjunction with terms like neural network and machine learning, highlighting OCT's role as a primary diagnostic tool in the field.
- Disease-Specific Terms: certain conditions, such as age-related macular degeneration and diabetic retinopathy, appear consistently over the years. These diseases are prominent applications for AI, as they benefit from automated screening and diagnostic support, particularly in regions where ophthalmic expertise may be limited.
This title-based analysis shows how research focus areas have remained relatively stable yet reflect nuanced shifts as new techniques and applications emerge within AI and ophthalmology.
Click me for more details
Evolution of Keywords and Key Phrases in Research Paper Abstracts
Loading plot...
This second stacked bar chart reveals the top 15 n-grams found in the abstracts of the "ophthalmology", "artificial", "intelligence" query we ran in PubMed. This view offers a more granular view of the topics covered since abstracts generally provide more detailed context compared to titles, allowing us to observe specific methodologies and metrics.
Notable observations include:
- Performance Metrics: terms such as sensitivity specificity and 95 CI (confidence interval) are frequently mentioned, especially in recent years. Such trends indicate an increasing emphasis on the statistical evaluation of AI models, reflecting the maturing focus on clinical validation and performance assessment.
- Imaging Modalities: alongside OCT, terms like fundus images are commonly cited, emphasizing the variety of imaging techniques being utilized. The occurrence of these terms in both titles and abstracts highlights the central role of imaging in AI-driven ophthalmology research.
- Neural Network and Deep Learning Techniques: the continued presence of terms related to neural networks and deep learning confirms the ongoing reliance on these models. However, the abstracts indicate a more detailed exploration of these technologies, with terms that delve into specific aspects of neural network architecture and application.
Over time, we can observe that while high-level themes like artificial intelligence remain stable, the details of implementation, such as specific metrics and imaging types, reveal an evolving landscape. As researchers continue to validate AI applications in ophthalmology, the field is steadily moving from exploratory studies towards clinically validated and deployable solutions.
Key Contributors to AI Ophthalmology Research Authors, Countries, Institutions, and Languages
Loading plot...
To gain a deeper understanding of the collaborative landscape in AI ophthalmology research, I mapped out the relationships among the most prolific authors, their affiliated countries, primary institutions, and the languages in which their work was published. The resulting visualization is presented in the Sankey chart above, which allows us to trace the flow of research contributions from individual authors through to the broader context of their institutions, countries, and languages.
The Sankey chart highlights the top five authors based on the number of publications within our dataset. Each author is connected to their country, indicating where the majority of their research was conducted or affiliated. From there, we can follow the flow to see the institutions associated with each author's work and, finally, the language in which their research was published.
This visualization not only reveals the primary contributors to AI in ophthalmology but also showcases the collaborative networks and institutional connections that support this research.
Main Observations:
- Author-Country Affiliations: for instance, Pearse A. Keane, a leading figure in this field, is associated with the United Kingdom and prominent institutions like the Moorfields Eye Hospital and University College London. His research reflects the strength of AI and ophthalmology collaborations within the UK.
- International Collaborations: Aaron Y. Lee’s work predominantly ties him to the United States, with connections to major research hubs such as the University of Washington and the National Institutes of Health. This highlights the significant U.S. contributions to AI in ophthalmology and suggests that much of this work is tied to major research centers with dedicated funding for health informatics and precision medicine.
- Diversity of Institutions: we see a variety of institutions represented, from high-profile hospitals and research centers in the U.S. and the UK, to specialized ophthalmic centers in China, such as the Zhongshan Ophthalmic Center. This diversity reflects the global interest and investment in AI-driven ophthalmology research.
- Language Distribution: while the majority of the research is published in English, reflecting the international standard in scientific communication, we can also observe minor flows to other languages, depending on regional institutions and author affiliations. This reaffirms the dominance of English in this research field but also hints at the presence of non-English contributions that could be expanded for a more inclusive knowledge base.
The Sankey chart provides a visual pathway through the networks of collaboration that drive AI in ophthalmology. By observing these connections, we gain insight into the influential research hubs, prominent figures, and the global nature of this field. This visualization underscores how AI advancements in ophthalmology are not isolated efforts but rather the result of a complex web of cross-institutional and cross-national collaborations.
Sure enough, the chart highlights how a small number of highly influential authors are connected to major institutions, underscoring the role of established research centers in the advancement of AI applications in ophthalmology. These connections also suggest potential areas for future collaboration, particularly in regions that are not currently as heavily represented, to further expand the field's reach and impact.
Value-Based Health Care (VBHC)
Problems Facing Healthcare Delivery Organizations
I would like to conclude this presentation by considering the broader implications of the use of artificial intelligence within ophthalmology as it relates to healthcare providers ability to deliver improved patient outcomes.
Increasingly, national governments and healthcare providers have struggled to respond to the dynamic global healthcare landscape, where a notable increase in demand has been driven by, amongst other causes:
- An aging population.
- Growing affluence.
- A surge in the treatment of chronic illnesses.
- A more knowledgeable patient population.
As a result, the quality of care administered by healthcare networks is at great risk, costs are likely to (continue to) rise, and patients become increasingly unsatisfied with their healthcare options. All the while there remains a significant misalignment between healthcare supply and demand.

Although older technology systems are beginning to be updated, they have yet to catch up with patient expectations. The introduction of electronic medical record (EMR) solutions has provided some improvements, but many technology platforms still need upgrades.
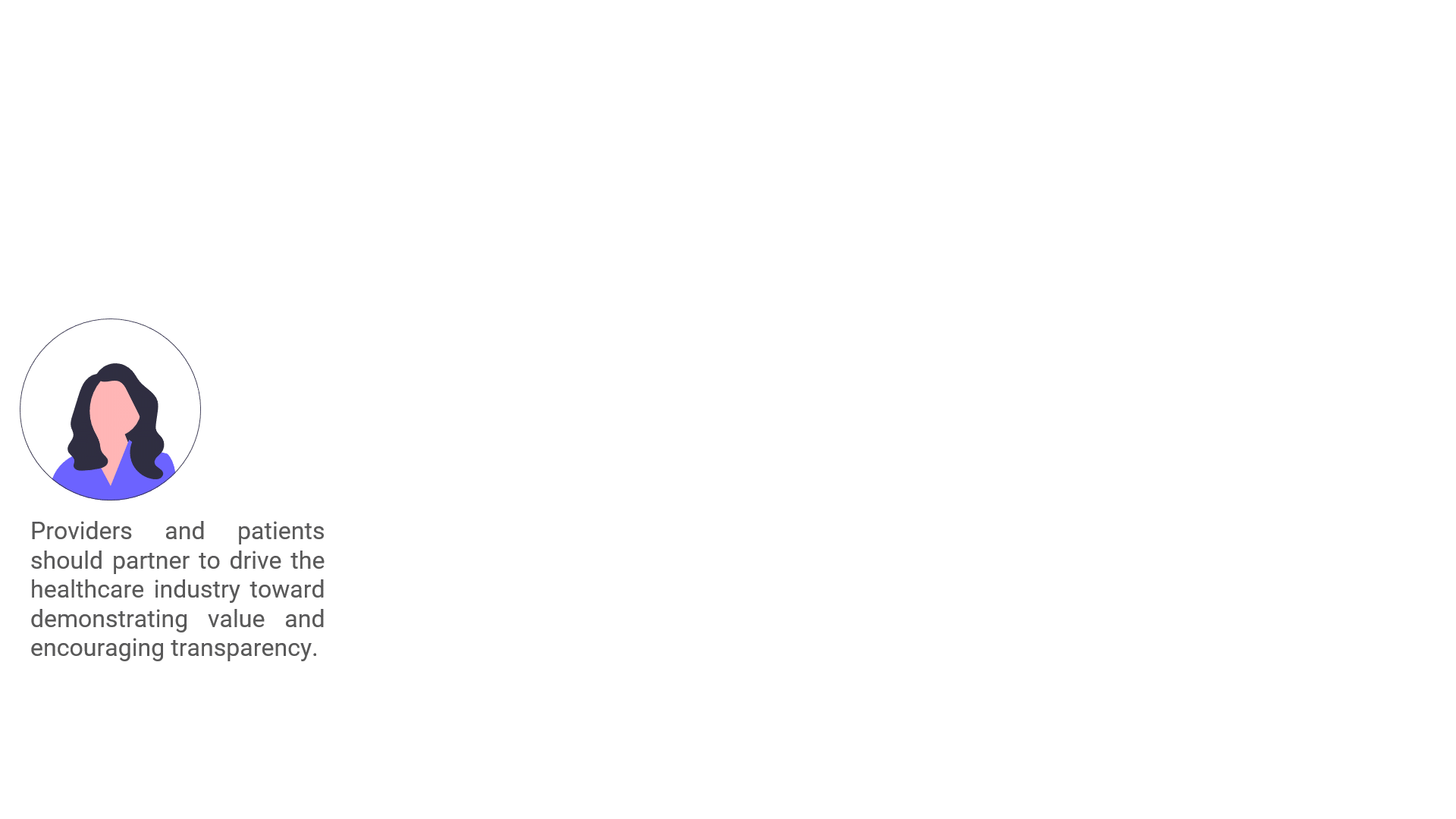
Recommendations for Equity in Healthcare Delivery

Where providers in the ophthalmology space are implementing artificial intelligence solutions into their daily clinical practice, or examining new healthcare delivery stategies that prioritize value over volume, there comes hand-in-hand the opportunity to do so alongside a commitment to adopting a value-based healthcare model.
Value-based healthcare (VBHC) is a model of healthcare delivery that seeks to improve patient outcomes by realigning the focus of healthcare providers from the volume of services provided to the value created for patients. It proposes that improving value requires either improving one or more outcomes without raising costs, or lowering costs without compromising outcomes, or both (Porter, M. E. and Lee, T. H., MD. 2013). The VBHC model is designed such that providers can guarantee access, for all, to the state of the art in healthcare.
We are very much experiencing a transition to a fourth industrial revolution; that of artificial intelligence. While it may seem that this technology is magical, it is more grounded to assert how clearly it has the potential to improve all of our lives, especially when applied in medicine and healthcare.
But with this being the case, artificial intelligence is a technology that should be open to everyone. And this openness can only be achieved through shared access to resources. In particular, access to the enormous amounts of data needed to train and teach AI algorithms. And an understanding of the north-south hemisphere divide that the present work has highlighted. We have seen how wealthier countries have the power to steer the direction of the research landscape. But in doing so they can dictate which topics are investigated and which are ignored.
Only through equitable access to this technology and the underlying data can we ensure that the age of AI is available to all.
Conclusions
This exploration into the intersection of artificial intelligence and ophthalmology has provided a panoramic view of the current research landscape, revealing both the promise and the challenges inherent in this rapidly evolving field. By conducting a broad-based search through PubMed, we captured a high-level snapshot of nearly 4,000 research articles, each contributing a piece to the larger puzzle of how AI is being utilized to advance ophthalmic care.
Our initial analysis exposed a global imbalance in research contributions, with a significant portion of studies emerging from wealthier nations in the Northern Hemisphere. This disparity raises pressing questions about equitable access to AI advancements in healthcare, particularly for lower-income countries in the Southern Hemisphere. The dominance of English-language publications further underscores the challenges faced by non-English-speaking regions in accessing and contributing to the body of scientific knowledge. These observations highlight a critical need for more inclusive practices in the dissemination of AI research, so that its benefits can extend to underserved regions worldwide.
Through advanced topic modeling using BERTopic, we delved deeper into the thematic content of the research corpus. The resultant visualizations - both 2D and interactive 3D scatterplots - unveiled a complex landscape of semantic relationships, where specific clusters reflect areas of concentrated research, such as retinal disease detection, robotic surgery, and AI-based diagnostic tools for retinopathy of prematurity. This navigable "map" of research topics provided a clear and nuanced view of the field's focal points and illustrated how AI's applications in ophthalmology are both diverse and interlinked.
The analysis of keywords over time showed a field in transition. As the titles consistently emphasized core topics like AI techniques and disease-specific terms, the abstracts revealed a growing sophistication in methodology, marked by detailed discussions of performance metrics and imaging modalities. This evolution from exploratory studies to clinically validated solutions suggests that AI in ophthalmology is progressing steadily toward practical, deployable applications that hold real promise for improving patient outcomes.
The Sankey chart highlighted the networks of collaboration that underpin AI in ophthalmology; showcasing the influential authors, institutions, and cross-national partnerships driving a range of advancements. This interconnected web of research hubs underscores the notion that progress in AI is rarely an isolated endeavor. Rather, it is built on a foundation of shared knowledge, resources, and institutional support that spans borders and disciplines.
Finally, in reflecting on the importance of value-based healthcare (VBHC), we are reminded of the broader context within which these AI technologies must operate. As healthcare systems around the world grapple with rising costs, resource constraints, and patient dissatisfaction, the VBHC model offers a path forward, emphasizing outcomes that matter to patients over the volume of services provided. For AI to fulfill its transformative potential in this context, access to the technology must be democratized. This includes open access to the vast datasets required to develop robust AI models and a concerted effort to bridge the North-South divide in research capabilities and resources.
In closing, the age of AI in ophthalmology holds extraordinary promise, but achieving its full potential will require a commitment to inclusivity, transparency, and collaboration. Only by ensuring equitable access to AI technology and the underlying data can we enable a future where the benefits of these advancements reach all corners of the globe, transforming ophthalmic care for patients everywhere.